As humans and robots work more closely alongside each other, the ability for both parties to understand and trust the actions of the other is likely to be crucial to effective collaboration. New research from the U.S. Army Combat Capabilities Development Command’s Army Research Laboratory suggests that human facial expressions could be crucial in establishing that trust, at least on the battlefield.
“We wanted to characterize and quantify factors that impact the emotional experience that humans have with trust in automated driving,” the researchers explain. “With this information, we want to develop a robust way to predict decision errors in automation use to eventually enable active, online mitigation strategies and effective calibration techniques when humans and agents are teaming in real-time.”
The researchers used flexible mixture modeling to sort participants into four groups based upon observed differences in traits, such as age, personality, and states (ie trust or stress). They then analyzed the facial recognition-based measures of emotional expression and self-report measures of trust under a range of different conditions of automation.
“It is often stated that for appropriate trust to be developed and effectively calibrated, an individual’s expectations must match the system’s actual behaviors,” the researchers explain. “This research shows that calibration metrics will vary across people; certain groups may be more prone to over-trust automation, while others may mistrust the automation from the start. From a research and development perspective, this approach provides a way to tailor human automation interaction modalities to individuals without needing to define and validate specific models for each person.”
Building trust
The researchers provide a number of recommendations for researchers when testing various interventions to help calibrate trust between mixed man and machine teams.
1) Researchers should not expect the same requirements for interventions for all individuals
2) Researchers can use multivariate methodologies focused on subgroups to identify clusters of individuals, which may inform expectations of varying responses and therefore directly influence selection of intervention strategies
3) Though often neglected in this literature base, researchers can use estimates of emotional expression (i.e., using facial recognition) for additional insights to improve trust calibration strategies
“Results showed that the group of individuals who were prone to over-trust also expressed strong outward changes in facial expression and were more likely to need interventions that directed their expectations and encouraged appropriate take-overs,” the researchers conclude. “Whereas a group with an inherent bias against automation had limited facial expression and may even be more negatively influenced by a similar type of intervention. Online mitigation strategies are unlikely to be robustly effective without accounting for this kind of inter-individual variability using these kinds of methods.”

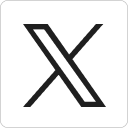



