Income inequality is sadly on the rise around the world, with the Covid pandemic exacerbating pre-existing issues, both on an international and national level. Many efforts to grapple with this challenge focus on the Gini coefficient, which measures resource distribution within a population. This metric scores perfect equality as zero, with maximum inequality scored as one.
While the Gini coefficient tends to dominate our thinking around inequality, research from Harvard suggests we can do better. They argue that while Gini does allow us to compare the concentration of income in various regions, it doesn’t always reflect how resources are distributed.
The depth of the problem
The researchers use trousers to illustrate the problem, as while picking a pair based on the waist size may broadly work, it’s likely to be better to choose based on the waist and leg measurements. Just as it’s good for retailers to devise measurements that reflect the diversity of people, so too should policymakers.
They compare 17 different measures and find that something known as the Ortega method, which comprises of two distinct variables, seems to be most effective when put to use on 3,000 or so different income distributions across the US.
The researchers explain that the Ortega parameters generally contain much more information than Gini captures, with each of the parameters focusing on a different aspect of income distribution. For instance, the first parameter captures the income distribution between low-income earners and medium-to-high-income earners. The second captures how the extremely wealthy compare to everyone else. The aggregate of both provides an inequality metric.
The researchers believe this approach captures different kinds of inequality, and importantly where the disparity comes from. They created interactive maps to allow us to dive into particular areas and gain such an understanding of local inequality.
Richer understanding
Using this approach provided the researchers with a much richer understanding of the problem. For instance, they found that obesity was not linked with inequality when using the Gini coefficient, but strongly correlated when using the Ortega parameters.
Similarly, the Ortega measures highlight the link between inequality and educational outcomes much more strongly than Gini does. This is particularly so in areas where inequality is driven primarily by the difference between low earners and medium-to-high earners, where we can expect a much lower share of degrees in the population.
This greater nuance will hopefully help policymakers deliver interventions that are more likely to succeed in changing the picture of inequality. These parameters may be used in other ways to drive a large number of other policy-relevant outcomes including initiatives related to health, crime, and social mobility.

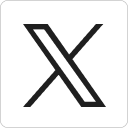



