A few years ago I wrote about research showing how biased stock images tend to be. This results in searches for “engineer” returning predominantly male images, and searches for “cleaner” returning predominantly female images.
Research from New York University highlights how harmful such biased search results can be. The study reveals that even gender-neutral internet searchers can still often yield male-dominated results, which can have a significant impact on hiring decisions and help to propagate gender biases.
“There is increasing concern that algorithms used by modern AI systems produce discriminatory outputs, presumably because they are trained on data in which societal biases are embedded,” the researchers explain. “As a consequence, their use by humans may result in the propagation, rather than reduction, of existing disparities.”
Spreading bias
In Equality Machine, the University of San Diego’s Orly Lobel that while we often focus on the negative aspects of AI-based technologies in spreading bias, they can also play a crucial role in making things better due to their ability to strip out the biases that are so difficult to strip out of human decision making. It’s a proposal shared by the NYU researchers.
“These findings call for a model of ethical AI that combines human psychology with computational and sociological approaches to illuminate the formation, operation, and mitigation of algorithmic bias,” they explain.
Many of the problems faced by AI systems today are due to the bias-infused data that they are trained on. Various examples exist to highlight this, such as Amazon’s famous algorithm into the best Amazon employees that was based on the primarily white, male workforce at the firm.
Ethical AI
To try and improve matters, the researchers conducted a number of studies to determine how the level of inequality in society itself transfers into the level of bias in what algorithms output, and from that, how exposure to this output influences decision makers to perpetuate the biases.
They began by gathering data from the Global Gender Gap Index (GGGI), which provides a ranking of gender inequality across around 150 countries. The Index covers a wide range of inequality metrics, including economic participation, health and survival, educational attainment, and political empowerment, with each country then given a “gender inequality” score.
They then attempted to evaluate the level of gender bias in search results and other forms of algorithmic output. They did this by examining words that should ordinarily provide an equal chance of referring to a man or a woman were assumed to be male by the algorithm. These include things such as “student” or “human”.
They conducted searches via Google for these phrases in the dominant local language for 37 different countries, with the results showing that the proportion of images with a male bias was higher in countries with higher levels of gender inequality, which suggests that the algorithms are tracking the gender bias in society more generally.
Influencing behaviors
The researchers then set out to understand whether exposure to these biased algorithms was enough to shape people’s perceptions, and indeed even their decisions in ways that conform to pre-existing inequalities.
They did this courtesy of a number of experiments whereby participants were shown Google image search results for four different professions, all of which were designed to be unfamiliar to the participants, including chandler and peruker.
The gender composition for each profession was determined according to the Google image results that were returned for the keyword “person” in nations with high global gender inequality scores, such as Hungary and Turkey, and also for those with low gender inequality scores, such as Finland and Iceland.
Each participant provided a so-called prototypicality statement regarding each profession before they began, which acted as a baseline for the subsequent experiment. This involved them being asked whether each profession was more likely to be a man or a woman. Consistently, the volunteers regarded each profession as being more likely to be male than female.
Gravitating to the norm
When asked the same question after being exposed to the image search results, the volunteers in the low-inequality conditions consistently reversed their male-biased prototypes in relation to their original baseline assessment. Those in the high-inequality condition typically maintained their male-bias, which was reinforced by the image search.
What’s more, in a subsequent experiment, this exposure bled into one’s hiring preferences. Participants were asked to rate the chances of men or women of being hired in each of the professions while also being shown images of two possible candidates (one man, one woman). When asked who they would hire, the exposure to the images produced more egalitarian decisions in low-inequality conditions and more biased outcomes in the high-inequality condition.
“These results suggest a cycle of bias propagation between society, AI, and users,” the researchers conclude. “The findings demonstrate that societal levels of inequality are evident in internet search algorithms and that exposure to this algorithmic output can lead human users to think and potentially act in ways that reinforce the societal inequality.”

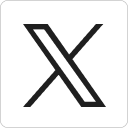



