The appearance of a new automobile holds far greater significance for automakers than just serving as a decorative cover for the engine. In fact, the design elements, including the proportions and colors of the body shape, as well as the intricate interplay between the headlights, grille, and bumper, which make up the vehicle’s “face,” together account for up to 60% of consumers’ purchasing decisions.
Research from Yale explores how AI can help to augment the design process and make some of the time- and cost-intensive aspects of vehicle design.
AI Assistance
The researchers developed a two-part machine-learning model that could revolutionize the way automobile designers and marketers approach vehicle development. Firstly, the model could give designers the ability to experiment with new design concepts more efficiently, while the second component could aid marketers in determining where to direct their focus.
Due to the significant expenses associated with producing a life-size vehicle model, A/B testing different prototypes is not a viable option for product and market researchers. Instead, “theme clinics,” involving large focus groups of highly-targeted consumers evaluating designs on paper, are relied upon. These clinics provide insights into how consumers perceive a design concept, such as whether it is viewed as “aggressive,” “modern,” or “luxurious,” and assist marketers in selecting a design that will resonate with their intended market segment.
However, given the time and resources required to develop and refine designs, the hundreds of theme clinics conducted each year across various products and market segments can cost around $100,000 each. As a result, companies end up spending vast amounts of money and manpower on designs that ultimately fail to connect with focus groups and are subsequently abandoned.
Making things better
To create the algorithm, the researchers developed up a deep neural network that could determine how features of an image translate into consumer ratings. With 7,000 images of 203 vehicles and consumer ratings from focus groups that evaluated these vehicles, which had been provided by GM, the team could train the neural net. However, there were several obstacles to overcome.
Firstly, the dataset was considered too small to be reliable, so the researchers expanded it using a separate set of 180,000 unrated images. These helped the algorithm recognize various body shapes and learn how consumers respond to them.
The next challenge was to find a way to encode a large amount of data without losing key features, which the team solved using a novel encoding algorithm. As a result, their predictive model was able to make more accurate predictions than other state-of-the-art models, and the algorithm also generated new design concepts that could improve the vehicle design process.
The system was able to predict how consumers would rate designs 30-40% more accurately than state-of-the-art models, and to anticipate new design trends up to five years ahead of their release. It is worth noting, however, that the algorithm is not meant to replace human designers, but rather to help them explore more design concepts and generate new ideas more quickly.
“Design is a fundamentally creative process that both listens to the market but also endogenously guides the market itself,” the researchers conclude. “We are likely currently at the stage of AI where it is likely most effective to augment and improve how existing workflows are done, not replace them entirely.”

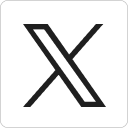



