The potential for artificial intelligence to bake in many of the biases us humans sadly maintain is one of the key challenges of AI today, and this was exemplified by new research from the University of California, Berkeley, the University of Chicago Booth School of Business and Partners HealthCare in Boston, which showed that a commonly used algorithm used to determine patients most at risk, and therefore most in need of urgent attention, would often discriminate against people of color. Just by fixing this one algorithm, the researchers believe that it would double the number of black patients who would be admitted to such programs.
“We found that a category of algorithms that influences health care decisions for over a hundred million Americans shows significant racial bias,” the researchers explain. “The algorithms encode racial bias by using health care costs to determine patient ‘risk,’ or who was mostly likely to benefit from care management programs.”
When the algorithm was tweaked so that it incorporated other variables, then the bias was largely corrected. It nonetheless underlines the relatively simple way in which algorithms can perpetuate biases if the data they’re fed on is not robust.
Regular audits
The researchers believe that regular audits of algorithms and the data they rely upon are crucial to prevent this from happening. Of course, when the AI has been developed by companies and therefore are proprietary, this is often easier said than done, especially by impartial parties.
The researchers attempted to overcome this by assessing around 50,000 patients at an academic hospital that was using a risk-based algorithm to determine whether patients were getting fair access to the hospital’s high-risk care management program.
The team got hold of the algorithm behind the program and were able to compare it alongside more direct measures of a patient’s health, such as the number of chronic illnesses they had. The analysis revealed that at certain risk scores, black patients had significantly worse health than their white peers.
“Instead of being trained to find the sickest, in a physiological sense, [these algorithms] ended up being trained to find the sickest in the sense of those whom we spend the most money on,” the researchers explain. “And there are systemic racial differences in health care in who we spend money on.”
High risk
Ordinarily, those with risk scores in the top 97% were automatically enrolled in the care management program, and with the simple intervention implemented by the researchers, the percentage of black patients falling into this automatic group leaped from just 18% to 47%.
By training the algorithms to better determine risks based upon a wider range of variables, the team believe that the chances of biases infecting the outcomes reduces considerably. Indeed, this was something the software developers behind the application took on board and are actively working to improve their program.
“Algorithms can do terrible things, or algorithms can do wonderful things. Which one of those things they do is basically up to us,” the researchers conclude. “We make so many choices when we train an algorithm that feel technical and small. But these choices make the difference between an algorithm that’s good or bad, biased or unbiased. So, it’s often very understandable when we end up with algorithms that don’t do what we want them to do, because those choices are hard.”

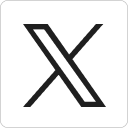



