Few of us actively enjoy having injections, yet for diabetics it’s a daily chore as they monitor their glucose levels. The daily finger prick can be sufficiently uncomfortable as to prevent people from keeping up with their daily routine, which imperils their health.
New research from the University of Warwick aims to allow people to monitor their glucose without requiring any needles. The technology utilizes AI to detect hypoglycemic events after monitoring the ECG signals produced by wearable devices.
At the moment Continuous Glucose Monitors (CGM) for hypoglycemia measure glucose in blood via a sensor with a small needle that sends data to a display device. The devices work well, but typically require daily calibration via finger-prick blood glucose level tests.
Automatic detection
The research highlights how AI can be trained to detect potential hypoglycemic events directly from within raw ECG data, all whilst using noninvasive wearable sensors bought off-the-shelf.
When the method was tested, the system achieved average sensitivity and specificity of around 82%, which is comparable to current rates of hypoglycemia detection in CGM systems.
“Fingerpicks are never pleasant and in some circumstances are particularly cumbersome. Taking fingerpick during the night certainly is unpleasant, especially for patients in pediatric age,” the researchers say. “Our innovation consisted in using artificial intelligence for automatic detecting hypoglycemia via few ECG beats. This is relevant because ECG can be detected in any circumstance, including sleeping.”
The model was capable of highlighting the ECG changes in each individual as the hypoglycemic event unfolded. The system was trained using data from each individual, with the researchers accepting that sufficient differences between people mean that relying on standardized data would not work. As such they hope their system will result in more personalized therapeutic solutions emerging.
“The performance of AI algorithms trained over cohort ECG-data would be hindered by these inter-subject differences,” the authors conclude. “Our approach enables personalized tuning of detection algorithms and emphasize how hypoglycemic events affect ECG in individuals. Basing on this information, clinicians can adapt the therapy to each individual. Clearly more clinical research is required to confirm these results in wider populations. This is why we are looking for partners.”

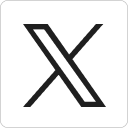



